simulx(): simulation of mixed effects models and longitudinal data
Description
Compute predictions and sample data from Mlxtran
and R
models
Usage
simulx( model = NULL, parameter = NULL, covariate = NULL, output = NULL, treatment = NULL, regressor = NULL, occasion = NULL, varlevel = NULL, group = NULL, project = NULL, nrep = 1, npop = NULL, fim = NULL, saveSmlxProject = NULL, result.file = NULL, addlines = NULL, settings = NULL )
Arguments
model |
(string) a Mlxtran model used for the simulation. It can be a text file or an output of the inLine function. |
parameter |
One of
|
covariate |
One of
|
output |
output or list of outputs. An output can be defined by
|
treatment |
treatment or list of treatments. A treatment can be defined by
|
regressor |
treatment or list of treatments. A treatment can be defined by
|
occasion |
An occasion can be defined by
|
varlevel |
deprecated, use occasion instead. |
group |
a list, or a list of lists, with fields:
“level” field is not supported anymore in RsSimulx. |
project |
the name of a Monolix project |
nrep |
Samples with or without uncertainty depending which element is given as “parameter”. |
npop |
deprecated, set parameter = “mlx_popUncertainSA” or “mlx_popUncertainLin” instead. |
fim |
deprecated, set parameter = “mlx_popUncertainSA” or “mlx_popUncertainLin” instead. |
saveSmlxProject |
If specified, smlx project will be save in th path location (by default smlx project is not saved) |
result.file |
deprecated |
addlines |
a list with fields:
“section”, “block” field are not supported anymore in RsSimulx. You only need to specify a formula. The additional lines will be added in a new section EQUATION. |
settings |
a list of optional settings
|
Details
simulx takes advantage of the modularity of hierarchical models for simulating different components of a model: models for population parameters, individual covariates, individual parameters and longitudinal data.
Furthermore, simulx allows to draw different types of longitudinal data, including continuous, count, categorical, and time-to-event data.
The models are encoded using either the model coding language Mlxtran. Mlxtran models are automatically converted into C++ codes, compiled on the fly and linked to R using the RJSONIO package. That allows one to implement very easily complex models and to take advantage of the numerical sovers used by the C++ mlxLibrary.
Value
A list of data frames. Each data frame is an output of Simulx
Examples
Example 1: simulation from scratch
This example shows how to create a Simulation from scratch and plot the results.
myModel <- inlineModel(" [LONGITUDINAL] input = {A, k, c, a} EQUATION: t0 = 0 f_0 = A ddt_f = -k*f/(c+f) DEFINITION: y = {distribution=normal, prediction=f, sd=a} [INDIVIDUAL] input = {k_pop, omega} DEFINITION: k = {distribution=lognormal, prediction=k_pop, sd=omega} ") f <- list(name='f', time=seq(0, 30, by=0.1)) y <- list(name='y', time=seq(0, 30, by=2)) parameter <- c(A=100, k_pop=6, omega=0.3, c=10, a=2) res <- simulx(model = myModel, parameter = parameter, occasion = data.frame(time=c(0, 0), occ=c(1, 2)), output = list(f,y), group = list(size=4), saveSmlxProject = "./project.smlx") res <- simulx(model = myModel, parameter = parameter, occasion = data.frame(time = c(0, 0, 0, 0), occ1 = c(1, 1, 2, 2), occ2 = c(1, 2, 3, 4)), output = list(f,y), group = list(size=4)) res <- simulx(model = myModel, parameter = parameter, output = list(f,y), group = list(size=4)) plot(ggplotmlx() + geom_line(data=res$f, aes(x=time, y=f, colour=id)) + geom_point(data=res$y, aes(x=time, y=y, colour=id))) print(res$parameter)
Example 2: simulation from a Monolix project
This example shows how to simulate from a Monolix project (using a Monolix demo): first with all the simulation elements exported from Monolix (Simulx resimulates the dataset from Monolix using the population parameter estimated in the Monolix project), then with a new design for the treatment (4 doses are given to all individuals), output (Cc is recorded instead of CONC, so the residual error is not included in the simulations) and group size (20 simulated individuals).
# First use the Monolix connectors to get the path to the demos and estimate the population parameters of the Monolix project library(lixoftConnectors) initializeLixoftConnectors(software = "monolix") demoProject <- paste0(getDemoPath(),"/1.creating_and_using_models/1.1.libraries_of_models/theophylline_project.mlxtran") loadProject(demoProject) runPopulationParameterEstimation() # Then use RsSimulx for the simulations library(RsSimulx) res <- simulx(project = demoProject) plot(ggplotmlx() + geom_line(data=res$CONC, aes(x=time, y=CONC, colour=id)) + geom_point(data=res$CONC, aes(x=time, y=CONC, colour=id)))
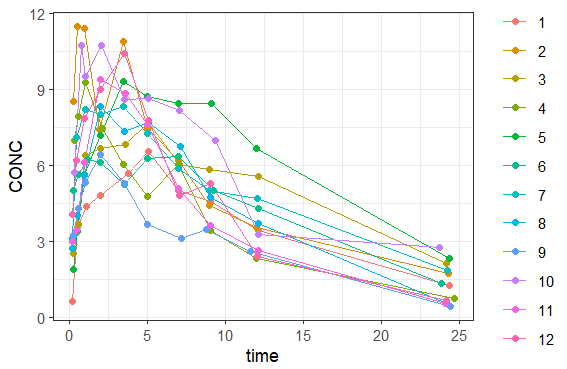
res <- simulx(project = demoProject, treatment = list(time=seq(0, by=24,length=4), amount=4), group = list(size=20), output = list(name="Cc", time=1:120)) prctilemlx(res$Cc)
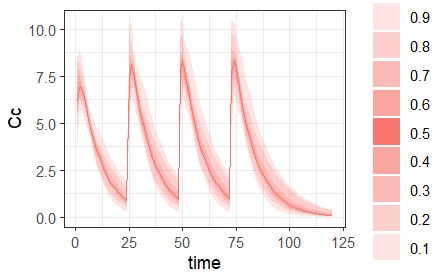